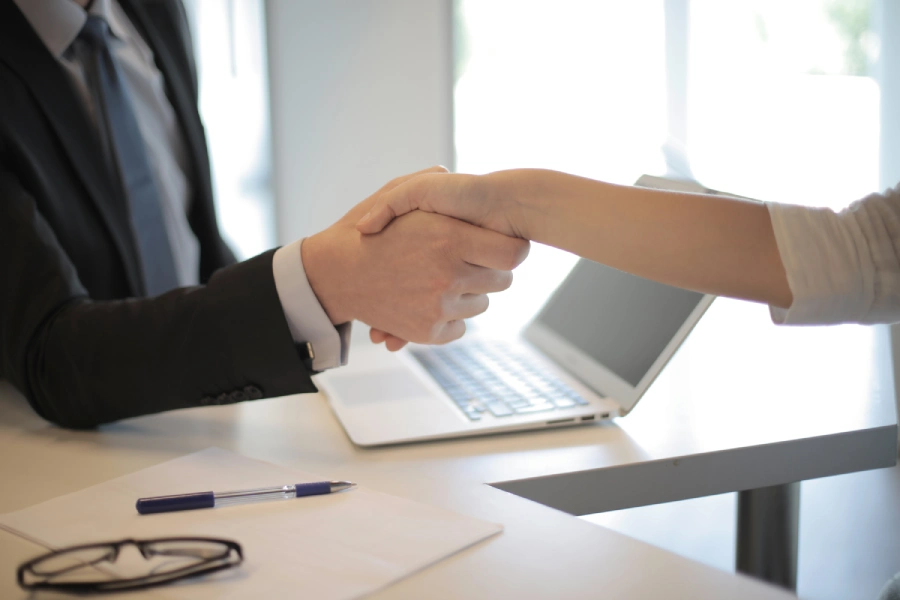
Optimizing Patient-Physician Matching: The Role of Predictive Analytics and Emerging Technologies
In the healthcare landscape, the patient-physician relationship is a critical determinant of medical outcomes and patient satisfaction. The traditional approach of assigning patients to physicians based on availability or geographical proximity is giving way to more sophisticated methods. These new methods leverage predictive analytics and emerging technologies to match patients with physicians based on patient profiles, demographic scores, and physician outcome scores. This blog post explores how these technologies can be used to optimize patient-physician matching and enhance healthcare outcomes.
Understanding Patient-Physician Matching
Patient-physician matching refers to the process of pairing patients with physicians who are best suited to meet their healthcare needs. This involves considering factors such as the patient’s health condition, preferences, and demographic characteristics, as well as the physician’s specialty, experience, and performance outcomes.
The Role of Predictive Analytics
Predictive analytics involves using statistical algorithms and machine learning techniques to identify patterns in data and predict future outcomes. In the context of patient-physician matching, predictive analytics can be used to analyze patient profiles and demographic scores, as well as physician outcome scores, to predict which physician-patient pairs are likely to result in optimal medical outcomes and patient satisfaction.
Patient Profiles and Demographic Scores
Patient profiles and demographic scores can provide valuable insights into a patient’s healthcare needs and preferences. These can include information about the patient’s health condition, age, gender, socioeconomic status, cultural background, and health behaviors. Predictive analytics can analyze these factors to predict the patient’s healthcare needs and preferences, and match them with physicians who are best equipped to meet these needs.
Physician Outcome Scores
Physician outcome scores provide a measure of a physician’s performance in terms of medical outcomes and patient satisfaction. These scores can be based on factors such as the physician’s success rate in treating certain conditions, patient satisfaction ratings, and adherence to clinical guidelines. Predictive analytics can analyze these scores to identify physicians who are likely to provide high-quality care and achieve positive medical outcomes.
Emerging Technologies in Patient-Physician Matching
In addition to predictive analytics, several emerging technologies can enhance patient-physician matching.
Artificial Intelligence (AI)
AI algorithms can analyze large volumes of data to identify complex patterns and make accurate predictions. In patient-physician matching, AI can be used to analyze patient profiles, demographic scores, and physician outcome scores to predict optimal matches.
Blockchain
Blockchain technology can provide a secure and transparent platform for storing and sharing patient profiles and physician outcome scores. This can facilitate the exchange of information between patients, physicians, and healthcare organizations, enhancing the efficiency and accuracy of patient-physician matching.
Conclusion
Predictive analytics and emerging technologies offer promising tools for optimizing patient-physician matching. By leveraging these technologies, healthcare organizations can enhance medical outcomes and patient satisfaction, contributing to the overall quality of care. As the healthcare landscape continues to evolve, these technologies will play an increasingly significant role in personalizing and improving healthcare delivery.
Sources
- Mehrotra, A., Forrest, C. B., & Lin, C. Y. (2011). Dropping the baton: specialty referrals in the United States. Milbank Quarterly, 89(1), 39-68.
- Bates, D. W., Saria, S., Ohno-Machado, L., Shah, A., & Escobar, G. (2014). Big data in health care: using analytics to identify and manage high-risk and high-cost patients. Health Affairs, 33(7), 1123-1131.
- Obermeyer, Z., & Emanuel, E. J. (2016). Predicting the future—big data, machine learning, and clinical medicine. New England Journal of Medicine, 375(13), 1216-1219.
- Jha, A. K., Orav, E. J., Zheng, J., & Epstein, A. M. (2008). Patients’ perception of hospital care in the United States. New England Journal of Medicine, 359(18), 1921-1931.
- Rajkomar, A., Dean, J., & Kohane, I. (2019). Machine learning in medicine. New England Journal of Medicine, 380(14), 1347-1358.
- Kuo, T. T., Kim, H. E., & Ohno-Machado, L. (2017). Blockchain distributed ledger technologies for biomedical and health care applications. Journal of the American Medical Informatics Association, 24(6), 1211-1220.